From Grocery Lists to Gothic Castles: Text-to-Image - AI's New Party Trick
- Priya
- Mar 6, 2024
- 6 min read
Updated: May 17, 2024
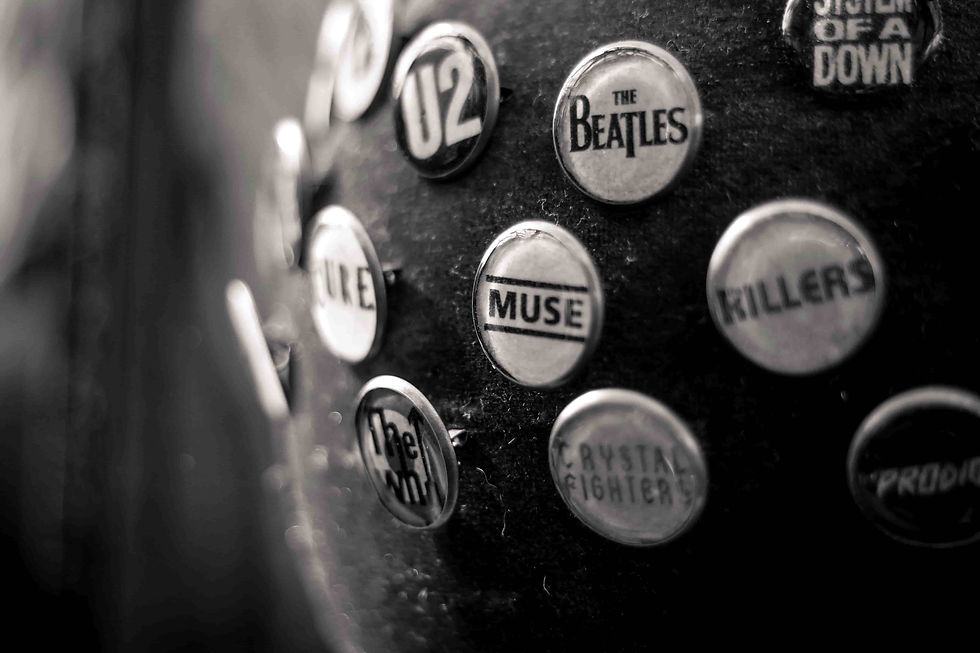
Imagine conjuring a vibrant picture with the mere power of your mind. Describing a scene in detail, and witnessing it come to life on a canvas. This captivating ability, once confined solely to the realm of imagination, is becoming increasingly achievable with the revolutionary field of text-to-image synthesis.
This technology, powered by generative models, unlocks a remarkable possibility: automatically generating images based on textual descriptions. It's like having a personal artist who interprets your words and translates them into visual masterpieces.
The Spark of Creation: Understanding Text-to-Image Synthesis
Text-to-image synthesis delves into the fascinating world of artificial intelligence (AI) and machine learning. At its core, the technology relies on models trained on massive amounts of data. This data consists of text-image pairs, where each pair includes a textual description and its corresponding image. By analyzing these paired examples, the models learn the intricate relationship between language and visual representation.
Think of it like teaching a language to a computer. Just as you learn the connection between words and their meanings, the model learns to associate specific words and phrases with visual elements like colors, shapes, and textures. Once trained, the model can then generate new images based on novel textual descriptions provided by users.
"Text-to-image models are like having a personal artist in your pocket, ready to bring your ideas to life with the power of words." - Jeff Clune, Chief Scientist at OpenAI
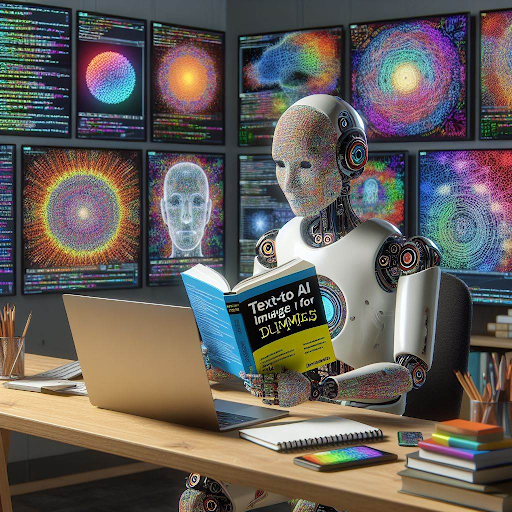
2022: A Year of Remarkable Advancements in Text-to-Image Models
2022 marked a significant year in the evolution of text-to-image models. These models, powered by artificial intelligence, can generate stunning images based on textual descriptions. This year witnessed a rapid pace of innovation, with new models like OpenAI's DALL-E 2, Google's Imagen, and StabilityAI's Stable Diffusion pushing the boundaries of what was possible.
Diffusion Models Take Center Stage:
Similar to the transformative impact of transformers in natural language processing, experts believe diffusion models will have a similar effect on text-to-image generation. These models, gaining prominence in 2022, work by gradually refining a random noise image into the desired final image based on the provided text description. This approach proved highly successful, with several notable breakthroughs occurring throughout the year:
April 2022: OpenAI unveiled DALL-E 2, showcasing its capability to generate artistic and photorealistic images based on text prompts.
May 2022: Google introduced Imagen, further pushing the boundaries of image quality and realism.
August 2022: StabilityAI released Stable Diffusion, another powerful diffusion-based model.
November 2022: NVIDIA joined the scene with eDiffi, a novel diffusion model raising the bar for text-to-image generation.
eDiffi: Redefining Text-to-Image Capabilities
While generating images from textual descriptions is impressive in itself, the latest research from NVIDIA with eDiffi takes things a step further. This innovative model introduces two groundbreaking features:
Combining Text and Reference Images: eDiffi allows users to incorporate a reference image alongside their text description. This is particularly beneficial for scenarios where complex concepts are difficult to describe solely with words. The reference image guides the model in applying the desired style to the generated image.
Paint-by-Word for Precise Control: eDiffi empowers users with granular control over object placement within the generated image. By associating specific phrases in the text prompt with different areas of a virtual canvas, users can dictate the layout and arrangement of objects in the final image.
These advancements in text-to-image models hold immense potential for various applications, from creative design and artistic exploration to education and scientific visualization. As research continues to accelerate, the future of this technology appears dazzlingly bright, offering exciting possibilities for generating even more sophisticated and user-controlled visual experiences.
The Artistic Palette: Exploring Different Generative Models
The world of text-to-image synthesis boasts a variety of generative models, each with its unique approach to the creative process:
Generative Adversarial Networks (GANs): Imagine two artists competing, constantly pushing each other to improve their work. This is the essence of GANs. They involve two neural networks: a generator that attempts to create realistic images based on the text description, and a discriminator that tries to distinguish between the generated images and real images from the training data. This continuous competition drives the generator to create increasingly realistic and accurate images.
Transformers: Inspired by the revolutionary architecture used in natural language processing (NLP), transformers excel at capturing the long-range dependencies within a sentence. This makes them well-suited for text-to-image synthesis. Transformer-based models learn to encode the textual description and then use this information to meticulously construct the image, pixel by pixel.
Diffusion Models: Picture a process akin to sculpting. Diffusion models begin with a random noise image and gradually refine it towards the final desired image based on the provided text description. Think of this as adding details and removing noise step-by-step, guided by the text instructions, until the final image emerges.
Each model has its own strengths and weaknesses, and the choice often depends on factors like the desired level of realism, the complexity of the description, and the computational resources available.
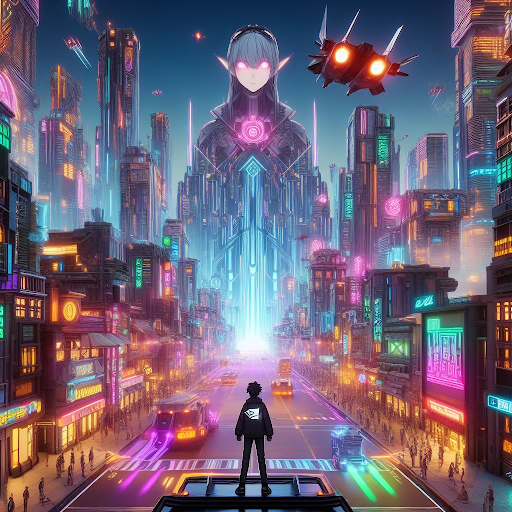
Beyond the Canvas: Challenges and the Road Ahead
While significant strides have been made, text-to-image synthesis still faces a few challenges:
Understanding complex semantics: Just like humans sometimes misinterpret nuances in language, models can struggle with complex or ambiguous descriptions. Ensuring accurate interpretation of intricate details remains a work in progress.
Generating diverse and creative outputs: While models can create realistic images, they sometimes lack the spark of true creativity. Encouraging models to generate diverse and original outputs that go beyond replicating existing styles is an ongoing pursuit.
Ethical considerations: The training data, and consequently the generated images, can inadvertently encode societal biases. Addressing these biases and ensuring fairness and inclusivity in the generated images is crucial.
Despite these challenges, researchers are actively working on addressing them, constantly refining model architectures, employing advanced training techniques, and fostering responsible AI development.
A Glimpse into the Future: Where Text-to-Image Synthesis Leads Us
The future of text-to-image synthesis is brimming with exciting possibilities:
Enhanced control and interpretability: Imagine being able to fine-tune the style, content, and composition of the generated images. This level of control and interpretability would empower users to create truly personalized visual experiences.
Multimodal learning: Combining textual information with other modalities like audio or video promises even richer and more immersive experiences. Imagine describing a bustling cityscape, and not only seeing the image but also hearing the sounds of traffic and conversations.
Real-time generation: The ability to generate images from text descriptions in real-time would open up a plethora of interactive applications. Imagine describing a new outfit for your character in a game and seeing it come to life instantaneously.
Text-to-Image: A Stepping Stone to a Broader AI Revolution
Large-scale diffusion models are spearheading a significant breakthrough in text-driven image generation, producing high-resolution images based on textual descriptions. This technology holds immense potential beyond just creating visuals from words.
Beyond Text-to-Image: A Ripple Effect on AI
The groundbreaking advancements in text-to-image models are merely the beginning. These advancements are expected to have a significant impact on other AI fields, leading to substantial improvements in both:
Computer Vision: Diffusion models offer the potential to generate vast amounts of realistic synthetic data, overcoming the limitations of collecting real-world data, which can be expensive and time-consuming. This synthetic data can be used to train computer vision models for various tasks, from medical image analysis to autonomous driving.
Natural Language Processing (NLP): The ability to generate textual descriptions of images can significantly improve NLP tasks like image captioning and visual question answering.
"It's fascinating to see how text-to-image models are blurring the lines between language and visual representation, opening doors to entirely new forms of creative expression." - Fei-Fei Li, Co-Director of the Stanford Human-Centered AI Institute
Language: The Bridge Between Our Minds and the World
Language plays a crucial role in shaping our world. We use it to describe and understand our surroundings, share knowledge across generations, and even explain the complex mental imagery we experience. Text-to-image models act as a bridge between the power of language and the visual world, opening doors to remarkable possibilities.
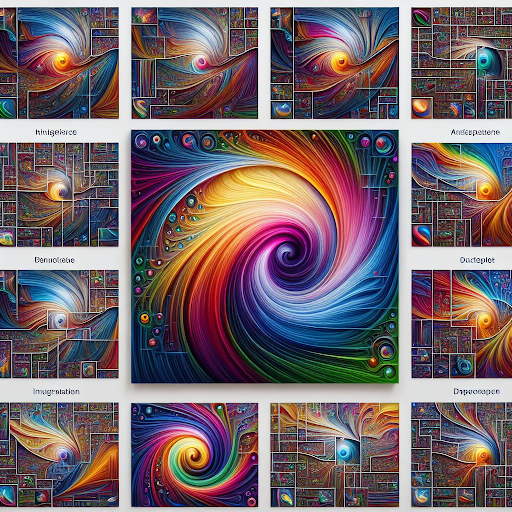
Real-World Applications of Text-to-Image:
The potential applications of text-to-image technology extend far beyond mere image creation. It can be used for various purposes across diverse industries, including:
Creative fields: Generating images for marketing campaigns, designing characters for games or animation, and creating unique artwork for NFTs.
Image restoration: Restoring blurry or low-resolution images and enhancing their resolution through super-resolution techniques.
Image editing: Filling in missing parts of images (inpainting) or making targeted edits based on textual descriptions.
In essence, text-to-image models mark a significant step towards a more advanced and versatile AI landscape. Their ability to bridge the gap between language and visual representation unlocks a plethora of applications across various domains, paving the way for a future filled with exciting possibilities.
Words Become Worlds: Witnessing the Rise of Text-to-Image, AI's New Storytelling Tool
The journey of text-to-image synthesis is like witnessing a seed blossom into a vibrant flower. From the initial spark of translating words into visuals to the current ability to generate high-fidelity images, the advancements in this field are truly remarkable.
This technology transcends the realm of mere image creation; it bridges the gap between language and visual representation, unlocking a treasure trove of potential across various domains.
Imagine a world where language becomes the brush and text descriptions transform into breathtaking visuals. Artists can explore uncharted creative territories, scientists can visualize complex concepts with ease, and everyday individuals can express their ideas in a whole new way. The possibilities are truly endless.
As we stand at the precipice of this exciting revolution, the future seems ablaze with the vibrant colors of imagination. While challenges remain, the relentless pursuit of innovation and the collaborative spirit of the AI community offer a glimpse into a future where words will not just paint a picture in our minds, but on the canvas of reality itself.
Ready to delve deeper into the captivating world of generative AI and explore its diverse applications? Follow TheGen.ai for Generative AI news, trends, startup stories, and more.
Commentaires